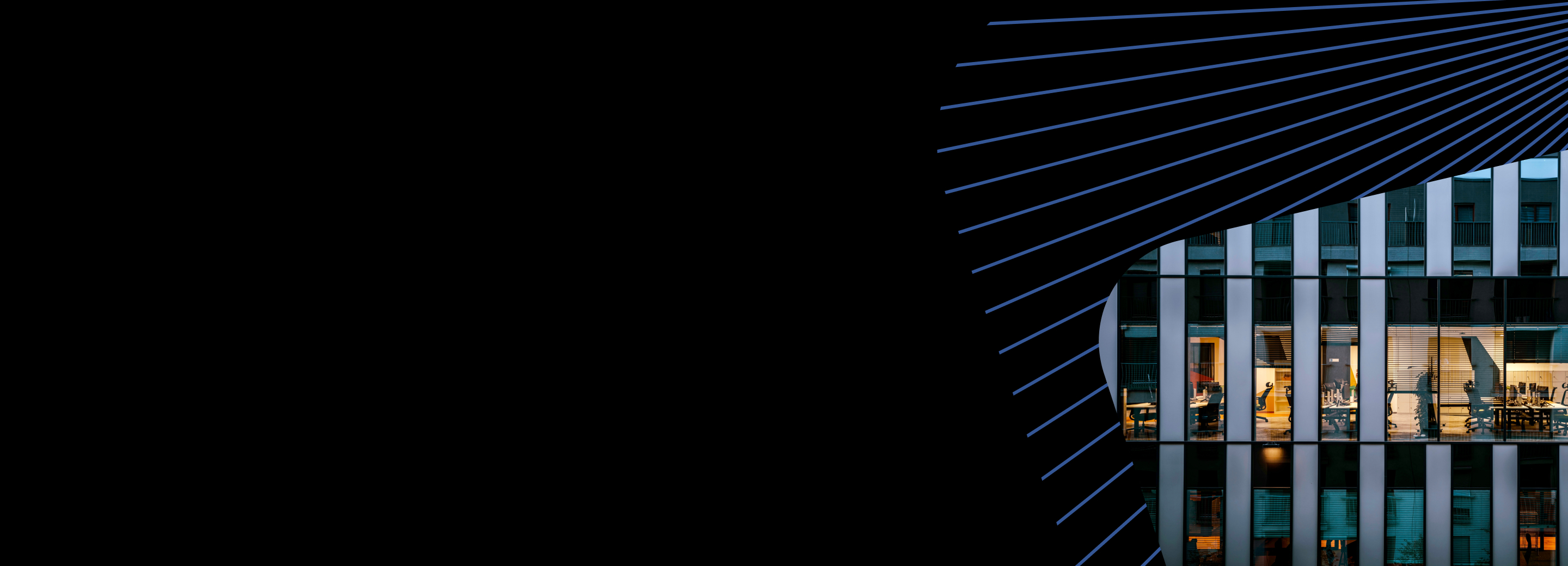
What’s A Data Mart?
Gain a comprehensive understanding of Data Mart, including its fundamentals and use cases for businesses. Learn how Data Mart can improve your analytics and boost business intelligence.
In a world where big data is transforming business and powering diverse technologies, Data Mart infrastructures play an important role in ensuring data is usable and accessible. The incredible amount of data available today makes it even more difficult to gather useful data and gain critical insights. This is why smart businesses invest in Data Marts. This ensures they have an aggregate amount of structured data in a centralized collection system for various business functions. This article explains the fundamentals of Data Marts and their use cases.
What is Data Mart? Meaning & Definition
Data Mart is known as a smaller, business, or team-specific subset of a bigger data warehouse. Data Marts are intended to give corporate users streamlined data access so they may research data trends and make wise decisions. Data Marts only store structured data from particular data sources, such as internal operational systems or external data sources, in contrast to data repositories or warehouses that store data in all forms.
A Data Mart’s function is to enable reporting and business intelligence by making specific data for a certain business unit or function quickly and easily accessible. Dimension tables and transactional data are kept in Data Marts, where they can be used to support decision-making, historical data analysis, and data mining. Data Marts can be utilized as an agile enterprise warehouse or as a helpful addition to an existing enterprise warehouses. However, to guarantee data integrity, reduce data redundancy, and maximize the utility of data stored in Data Marts, data governance is crucial.
Example
One example of a Data Mart is in sales, where there is a subset of a larger data warehouse that focuses on sales-related data. A sales store’s structured data is gotten from several data sources, including customer databases, point-of-sale systems, and CRM systems. Business users can quickly and easily access sales data from the Data Mart to analyze and make knowledgeable decisions. This system stores transactional data such as sales orders, invoices, and shipments.
Dimension tables for items, clients, and sales regions are frequently kept in sales Data Marts and can be used to facilitate data mining and historical data analysis. To guarantee data integrity, reduce data redundancy, and maximize the use of data housed in a sales Data Mart, data governance is essential.
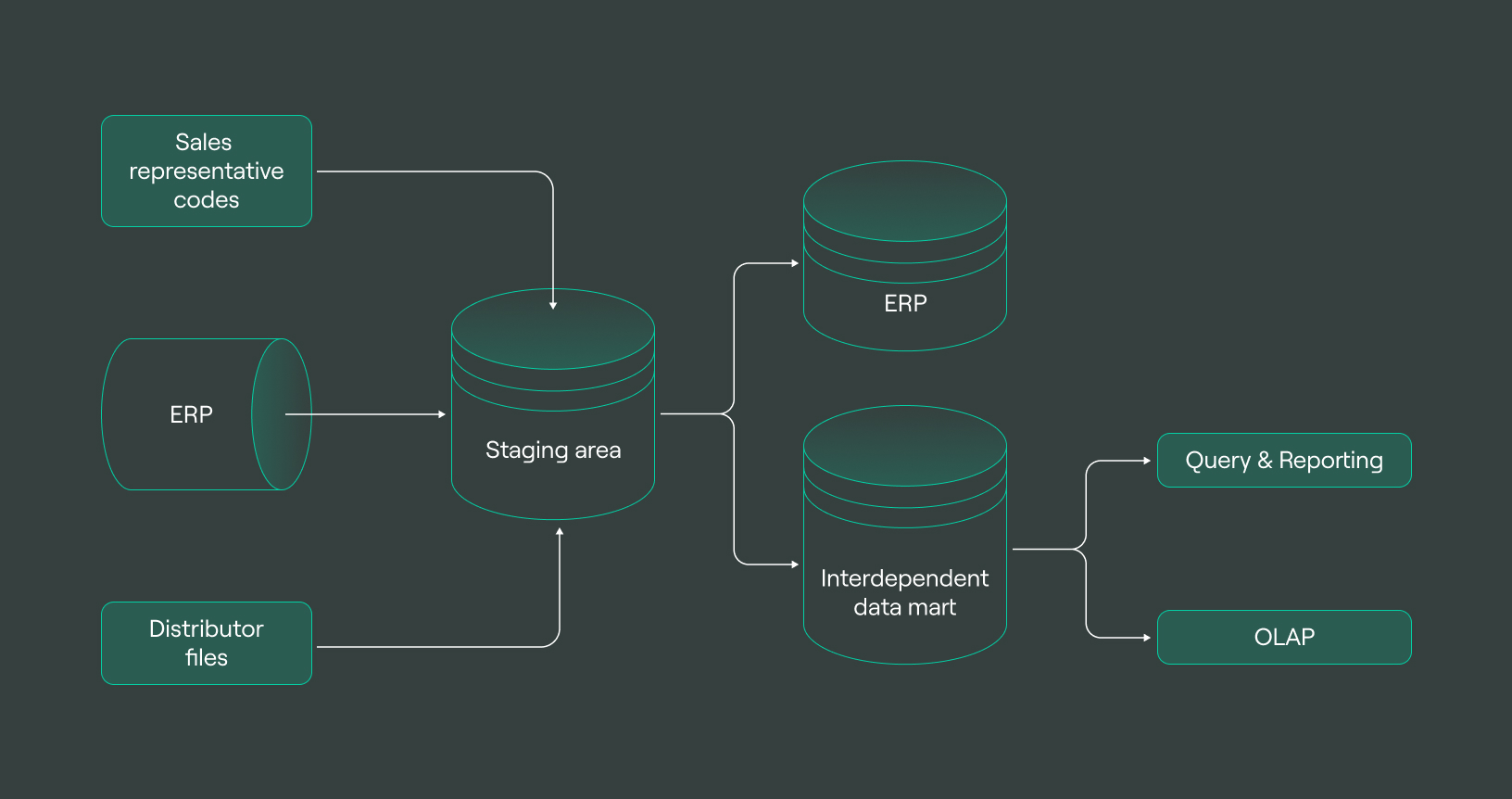
In this article, we’ll talk about:
- What is Data Mart? Meaning & Definition
- Why is a Data Mart important for businesses?
- How does a Data Mart work?
- Types of Data Marts
- Characteristics of Data Mart
- Data Mart Architecture
- Data Mart vs Data Warehouse: Is there difference?
- Who uses a Data Mart?
- The benefits of a Data Mart
- Data Mart Tools
- Challenges in Data Mart Implementation
- Best Practices for Data Mart Implementation
- Final words
Why is a Data Mart important for businesses?
Data Marts provide essential benefits for businesses as they provide a simplified and quick approach to accessing specific data, allowing business users to make well-informed decisions based on up-to-date information. By storing structured data from different sources in a separate Data Mart, businesses can streamline data access and minimize the complexity of querying data across its entire warehouse.
Data Marts enable businesses to focus on specific business functions or departments and generate tailored reports and analytics to facilitate business intelligence and enhance decision-making processes. Furthermore, Double.Cloud can help extract more insights from Data Marts by leveraging advanced analytics tools and machine learning algorithms to discover hidden patterns and relationships in the data. By utilizing the Double.Cloud platform, businesses can fully utilize their Data Marts and gain valuable insights that can help drive business growth and success.
How does a Data Mart work?
Data Marts are designed to provide quick and simple access to particular categories of data, such as sales, financial, or marketing data, allowing business users to evaluate and produce reports more quickly. The typical purpose of this data storage system is to hold structured data in a relational database, with fact tables containing transactional or historical data and dimension tables giving contextual information like date or customer.
By having independent Data Marts, businesses can avoid the complexity of querying data across an entire data warehouse and instead focus on specific areas of interest. Additionally, the Double.Cloud platform offers sped-up data access to Data Marts, allowing business users to derive insights from data using simple querying and visualization capabilities.
Types of Data Marts
There are three types of Data Marts which all possess distinctive characteristics that make them suitable for specific purposes.
Dependent Data Mart
A dependent Data Mart is one that draws its data sources from an existing data warehouse. It is designed to meet the demands of a particular department or business unit inside an organization by offering a subset of data that is tailored to the unit’s needs. Dependent Data Marts are generated utilizing the data that has already been extracted, transformed, and loaded (ETL) into the organization’s central data warehouse.
This is opposed to independent Data Marts, which can be developed without relying on an existing warehouse for enterprise data. Dependent Data Marts can be built more quickly and with less complexity, while still ensuring data integrity and consistency throughout the entire organization by utilizing the data stored in the warehouse. This is accomplished by using pre-built, warehouse-stored dimension tables that can be quickly accessed and used by the dependent Data Mart to generate unique reports and analyses.
Independent Data Mart
This is called independent specifically because it is made to meet the requirements of a particular department or business unit within an organization. Only the information relevant to that unit or department is contained in it, which was constructed separately from the main warehouse. Agile enterprise data warehouses benefit greatly from independent Data Marts because they can be designed and implemented more quickly than centralized ones.
The independent Data Mart, which can store transactional and historical data, is regularly updated. Users can access the data easily with simplified data access and querying data. The business unit can have more control over their data thanks to this kind of Data Mart, which also enables more focused data analysis to support business intelligence.
Hybrid Data Mart
A hybrid Data Mart is a combination of both independent and dependent Data Marts, allowing organizations to maintain a balance between flexibility and control. It is typically used when business units have specific requirements that cannot be met by the centralized data warehouse, but they still need to adhere to data governance policies. The hybrid data system takes advantage of the strengths of both independent and dependent Data Marts.
It provides agility and speed in data access like independent Data Marts while ensuring data consistency, data redundancy, and data integrity, like dependent Data Marts. This type of system allows organizations to efficiently manage and use their data by combining the benefits of both independent and dependent Data Marts, allowing for simplified data access, efficient querying of data, and support for business intelligence and data mining.
Characteristics of Data Mart
Data Marts possess different characteristics that show its type of design and how it works. The following are four characteristics that define Data Marts.
Subject-oriented
One of Data Mart’s defining features is its subject-orientedness. As opposed to a traditional data warehouses, is created to specifically address the requirements of a given business unit or function. Instead of being organized around the needs of the enterprise as a whole, the data is usually organized around a particular subject area, such as sales or inventory. Business users can now more quickly and easily access the data they require without having to wade through extraneous information.
The information in a Data Mart is typically obtained from a central warehouse or other data sources, but it is arranged and displayed in a manner that is specific to the particular business unit or function. In addition to lowering the risk of data redundancy and enhancing data governance, this helps to ensure that the data is accurate, consistent, and up-to-date.
Integrated
A Data Mart’s ability to integrate data from various sources to produce a single, comprehensive view of information is referred to as its integrated characteristic. As a result, the information kept it is not only subject-oriented but also combined from a variety of sources to give users a thorough understanding of a particular business function or process. Data from both internal and external sources, such as data lakes, relational databases, and business intelligence tools, are incorporated into the process of integration.
Data Marts give business users a centralized location to access data that is consistent, accurate, and up-to-date by combining data from various sources. By integrating these systems, data provided is guaranteed to be accurate and usable for data mining and querying, enabling businesses to base decisions on specific data trends and insights.
Time-variant
The 'Time-variant' characteristic refers to how data changes over time. In a Data Mart, data is stored in a way that allows for analysis of trends and patterns over time. This means that the data stored in a marts is time-stamped, enabling business users to query and analyze historical data to identify trends and patterns in the data. By capturing changes in data over time, Data Marts provide insights into how business data has evolved, which can help organizations make data-driven decisions.
Additionally, the time-variant nature of Data Marts allows businesses to track changes in specific data over time, which is particularly useful for tracking trends in sales, customer behavior, and other business functions.
Non-volatile
Data Marts are non-volatile, meaning that once data is stored, it cannot be altered or updated. This characteristic ensures data integrity and consistency, which is crucial for business intelligence and decision-making. Unlike operational databases that are subject to frequent changes, Data Marts store historical data that is relevant to specific business functions or departments. This allows for simplified data access and querying, as well as faster response times when retrieving information.
Data Marts can be independent or part of an existing enterprise warehouse, depending on the organization’s data management system and business requirements. While Data Marts are focused on a specific subset of data, they can still access and incorporate external data sources to support data mining and provide a comprehensive view of business trends.
Data Mart Architecture
Understanding Data Mart’s Architecture will bring you closer to grasping the orientation and potential it can offer.
Here are known Data Mart architectural approaches.
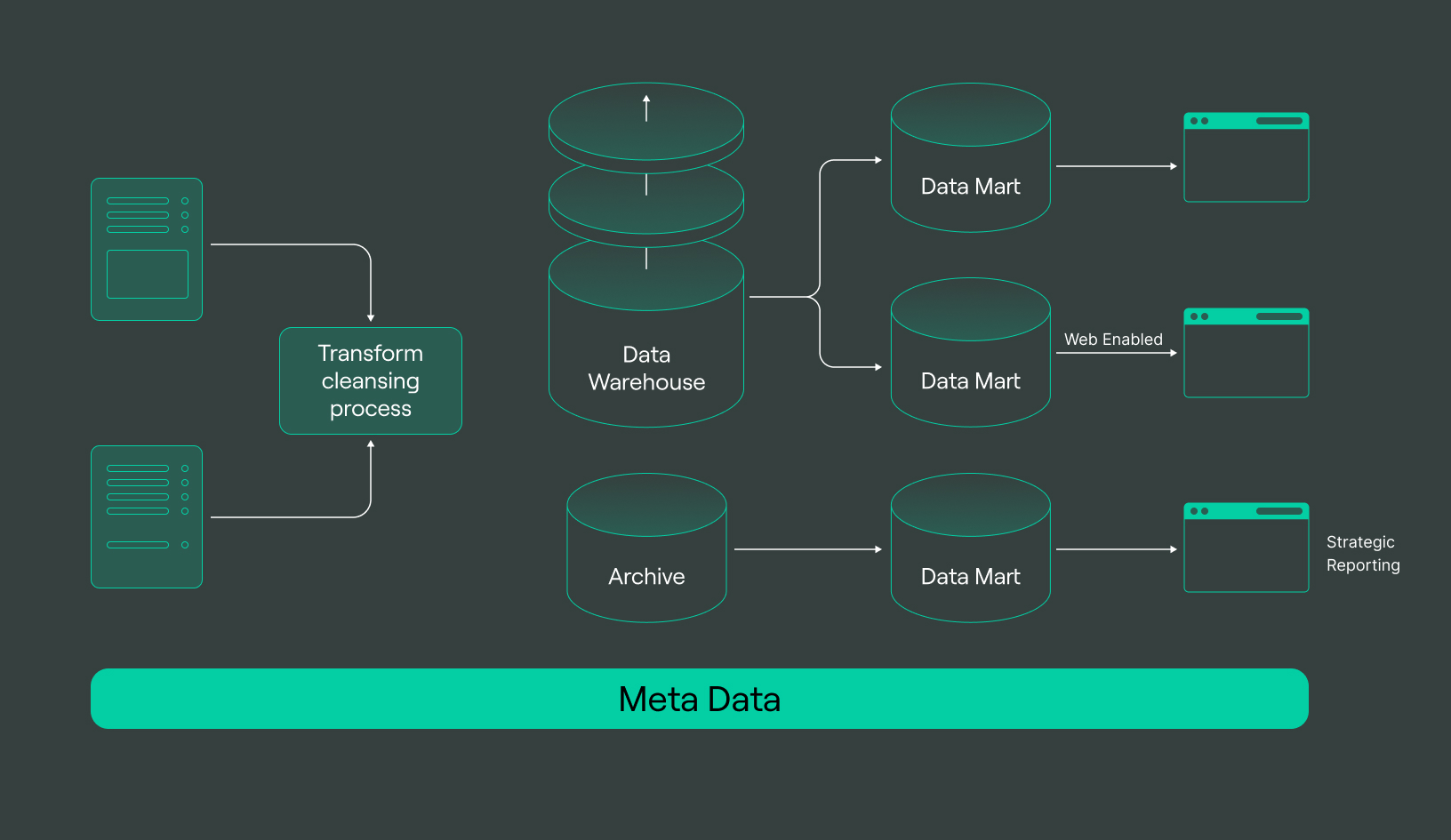
Bottom-up approach
In the bottom-up approach of Data Mart architecture, Data Marts are created from the operational data sources that a business unit or department uses. This approach starts with a department or business unit identifying the data they need to access to support their specific business function, and then creating a mart that stores that data. Data is loaded into the mart from internal operational systems or external data sources and then structured into dimension tables for simplified data access by business users.
This approach allows for a more agile warehouse, as it is built incrementally from specific data needs rather than attempting to design and implement an entire data warehouse upfront. Additionally, the use of independent Data Marts can provide faster querying of data and support data mining for specific data trends.
Top-down approach
The top-down approach to Data Mart architecture involves the creation of a centralized data warehouse, which serves as the primary data source for all Data Marts. In this approach, Data Marts are designed to serve the needs of specific business units or functions and are created based on the requirements of business users.
The data warehouses are designed to store all types of data, including structured and unstructured data, from various data sources, including internal operational systems and external data sources. Dimension tables and fact tables are used to store and organize data within the warehouse. The top-down approach to Data Mart architecture is a more warehouse-focused approach and is often used in larger organizations with more complex data requirements.
Federated approach
A federated Data Mart architecture is a data management approach that allows for the autonomous integration of multiple Data Marts while maintaining their independence. This approach enables organizations to access and analyze data from disparate sources without having to physically move the data in a centralized repository. Usually, in marts that have federated architecture, data remains in its original location and is accessed on an as-needed basis through a virtual layer.
This type of architecture provides increased agility and flexibility, as well as a simplified data access process, making it easier for business users to query data and gain insights. Additionally, a federated approach can support a variety of data types, including structured, unstructured, and semi-structured data, sourced from internal operational systems or external data sources, making it a versatile solution for businesses looking to streamline their data management processes.
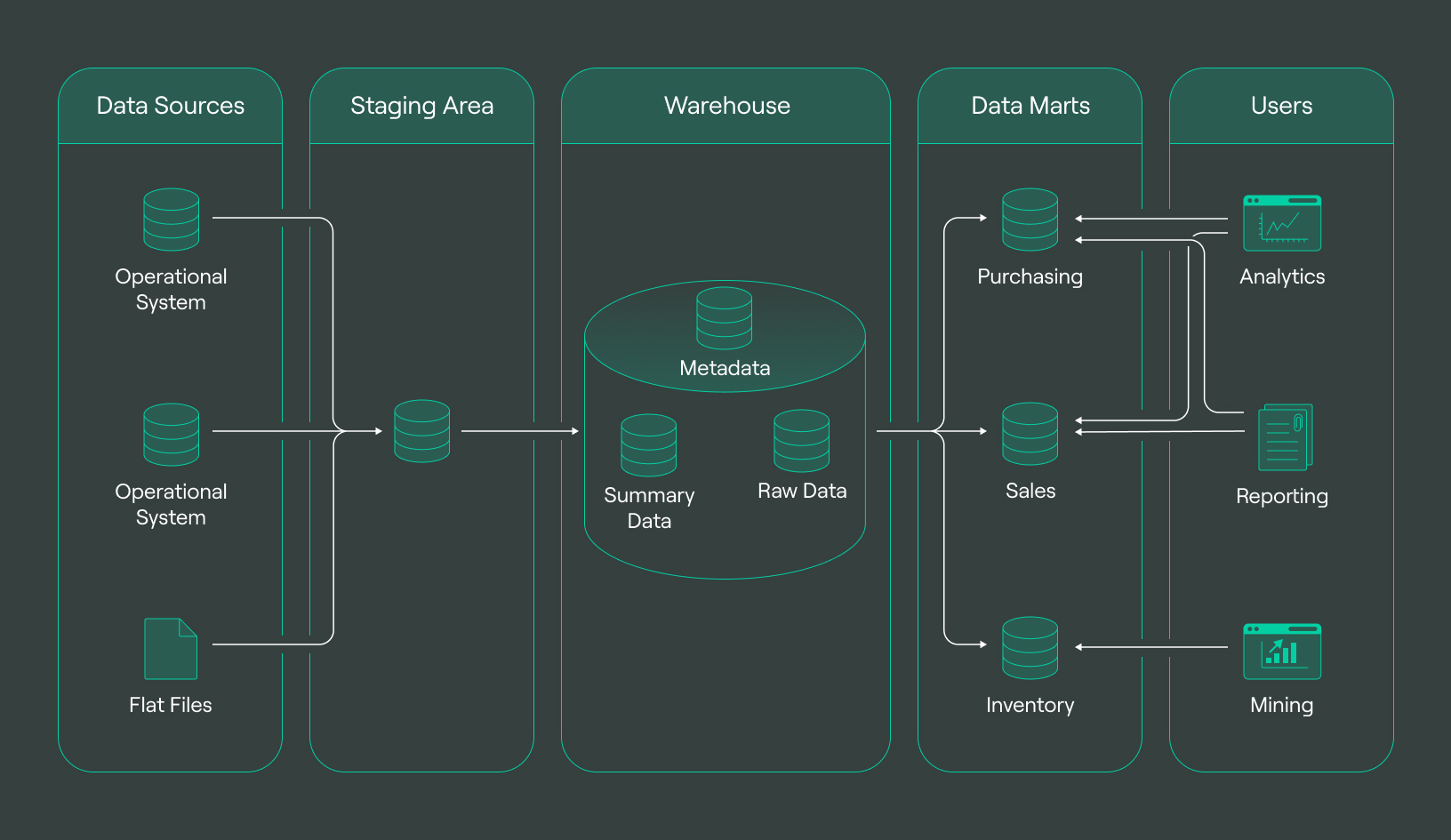
Data Mart vs Data Warehouse: Is there difference?
Data Marts and data warehouses are both key components in a business intelligence architecture that helps business users access and analyze data efficiently. While Data Marts are smaller, independent subsets of an organization’s data warehouse that focus on a specific business function or department, the warehouses are centralized repositories that store all of an organization’s data, including historical data from internal operational systems and external data sources.
Both Data Marts and warehouses store structured and unstructured data, including transactional data and raw data. However, Data Marts are designed to support a particular business function or specific data trend, while data warehouses are more data-focused and support data mining and querying data across the entire organization.
Difference |
Data Mart |
Data Warehouse |
Purpose |
Contains a subset of data for a specific business unit or function |
Stores all enterprise data for the organization |
Scope |
Narrow |
Wide |
Data Volume |
Small |
Large |
Data Granularity |
Fine |
Coarse |
Data Aggregation |
Summarized |
Detailed |
Data History |
Limited |
Extensive |
Data Source |
Specific to a business unit or function |
Multiple sources across the organization |
Data Governance |
Independent from the central data syste |
Governed by the central warehouse |
Data Integration |
May or may not be integrated with other Data Marts |
Integrated with other data sources and Data Marts within the data warehouse |
User Access |
Limited to specific business units or functions |
Accessible to all business units and functions |
Querying |
Optimized for specific business unit or function |
Optimized for complex queries and analytics |
Maintenance |
Less complex |
More complex |
Implementation |
Faster and easier |
Slower and more complex |
Who uses a Data Mart?
Typically, Data Marts are used by business users who need to analyze a specific set of data to support their decision-making processes. Data Marts are designed to be easily accessible, with simplified data access and querying capabilities, and they store a subset of data from the main data warehouse.
These independent Data Marts allow business users to quickly retrieve specific data without the need to navigate through the entire warehouse which can be overwhelming and time-consuming. For instance, a marketing team might use the data gathered to analyze sales data and customer demographics to identify trends and optimize their campaigns.
The benefits of a Data Mart
-
Easier Access to Data: Data Marts provide easier and quicker access to particular data subsets that are pertinent to a given business unit or function. They enable access to the data required by business users without requiring them to search through an entire data warehouse.
-
Better Query Performance: Since Data Marts are made to store a particular subset of data, queries run more quickly. The smaller size of Data Marts and their focus on a specific subject area are to blame for this improved performance.
-
Data Integrity: Data Marts are designed to guarantee data consistency and accuracy, and they aid in preventing errors brought on by redundant data. This promotes data integrity and guarantees that the data being used by business users is accurate and trustworthy.
-
More Flexibility: To accommodate a business unit’s or function’s changing needs, Data Marts can be easily built, modified, and maintained. This adaptability enables prompt adjustments to shifting business needs.
-
Cost-Effective: Data Marts are cost-effective because they are made to store a particular subset, which lowers data storage costs. Because Data Marts require less work than the entire warehouse, they are also more cost-effective in terms of design and maintenance.
Data Mart Tools
There are several tools available for managing and building Data Marts, including:
-
IBM InfoSphere Warehouse: This program offers data integration, data transformation, and data management functions, as well as data warehousing capabilities.
-
Oracle Database: The Oracle Data Warehousing option, which offers advanced data management, integration, and analysis capabilities, is one of the features for creating and managing Data Marts that are included in the Oracle Database.
-
Microsoft SQL Server: SQL Server Analysis Services, which offers multidimensional data analysis and reporting capabilities, is just one of the tools available through Microsoft SQL Server for creating and managing Data Marts.
-
Teradata: Teradata is a potent analytics and data warehousing platform that offers assistance with creating and maintaining Data Marts.
-
SAP BusinessObjects: This program can be used to create and maintain Data Marts because it has data integration, data quality, and data analysis features.
-
Tableau: To support business intelligence and decision-making, Tableau offers robust data visualization and analysis capabilities that can be used with Data Marts.
-
Amazon Redshift: An affordable and highly scalable cloud-based data warehousing solution, Amazon Redshift supports the creation and management of cloud Data Marts.
Depending on the unique requirements of the organization, each of these tools offers a variety of capabilities for creating and managing Data Marts, with varying strengths and weaknesses. To choose the right tool for a specific project or use case, it’s critical to carefully assess each one.
Challenges in Data Mart Implementation
Data Mart implementation can pose significant challenges in data integration, data quality, and data governance.
Data integration challenges can arise from inconsistencies in data sources, data structures, and data definitions.
Data quality challenges can arise from the lack of standardized data validation rules and procedures, leading to errors and inconsistencies.
Data governance challenges can arise from a lack of defined roles and responsibilities, leading to difficulties in maintaining data quality and ensuring data security.
These challenges need to be addressed through proper planning, effective data governance, and the use of suitable tools such as ETL (Extract, Transform, Load), data quality management, and metadata management tools.
Best Practices for Data Mart Implementation
The best practices for implementing this system involve several key steps:
-
Identify business needs and objectives: It is crucial to have a clear understanding of the business goals and objectives that the Data Mart will support. This will help ensure that the Mart is designed to meet the specific needs of the business.
-
Focus on data quality: High-quality data is essential for accurate reporting and analysis. Therefore, data quality should be a top priority throughout the implementation process.
-
Adopt a modular approach: Building the Data Mart in a modular fashion will make it easier to manage and maintain over time. This approach also allows for more flexibility and scalability as the business grows and changes.
-
Involve business users in the process: It is important to involve business users in the design and implementation of the Data storage Marts to ensure that it meets their needs and requirements.
-
Implement appropriate security measures: It should be designed with appropriate security measures to ensure that the data is protected and only accessible to authorized users.
By following these best practices, organizations can ensure that their Data Mart implementation is successful and supports their business objectives.
Final words
In conclusion, a Data Mart is a vital component of a business intelligence solution, serving as a subset of a data warehouse that is focused on specific business functions or departments. Its implementation requires a clear understanding of business objectives, a focus on data quality, a modular approach, involvement of business users, and the implementation of appropriate security measures. By adopting these best practices, organizations can leverage the benefits of Data Marts to make informed business decisions based on accurate and relevant data.
Frequently asked questions (FAQ)
Is data mart a database?
Is data mart a database?
Yes, a data mart can be considered a database. It is a subset of a larger data warehouse, designed to serve the needs of a specific business unit or function.
Why do businesses need data mart?
Why do businesses need data mart?
What is the purpose of a data mart?
What is the purpose of a data mart?
Get started with DoubleCloud
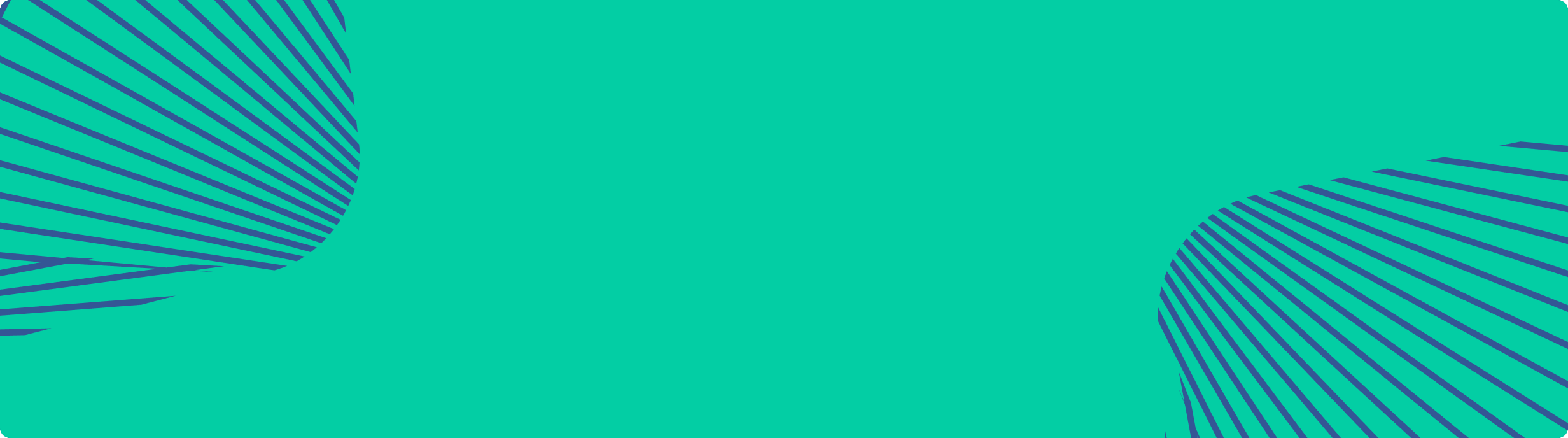