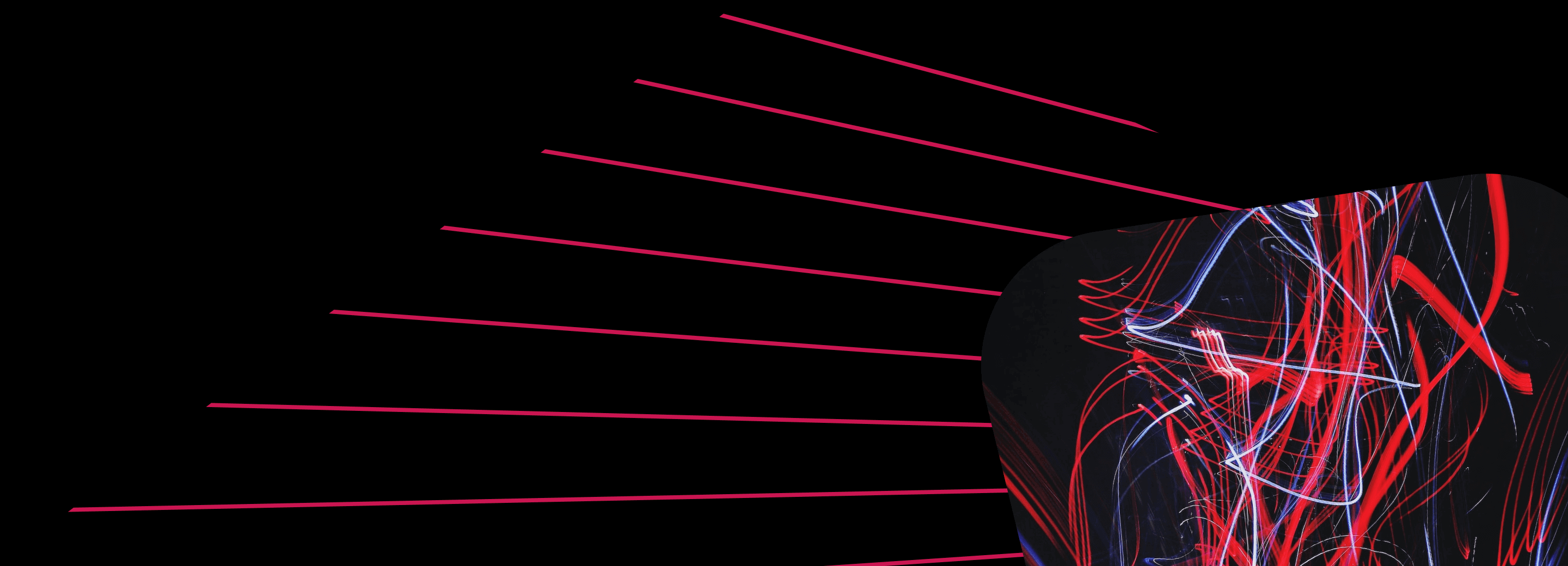
How to stop your data lake turning into a data swamp
In today’s modern world of data, the phrase Data Lake has become extremely common, allowing more and more organizations to store, retrieve and use the entirety of their data in an efficient and effective manner.
They’re a powerful tool in the Data Engineers kit, allowing them to store enormous volumes of multiformat, unstructured data whilst avoiding the need for silos.
But…
If your data team isn’t careful, a lake can very quickly become a swamp, and as you can imagine, a swamp is a difficult thing to traverse, much less do anything useful with.
Nothing permanent has ever been built on a swamp.
So, how do you stop your pristine and beautiful Data Lake turning into a Data Swamp?
First, let’s try and understand some of the terms involved…
What Is A Data Lake?
Data Lakes have been around for a few years now and, whilst they’ve become fairly standard, it’s slightly amusing to remember that when they first started appearing, many wrote them off as a marketing gimmick.
The problem was, that back when, Data Lake didn’t appear in any standard lexicon of data-storage terms or architecture — meaning it could (and did) mean different things to different people.
Fortunately (or we suppose unfortunately if your definition was one that fell by the wayside), the phrase has now become somewhat standardized, enough so that we can give a definition.
Data Lakes hold enormous amounts of unstructured (and often structured), raw or defined data in a native format with no schema for how it’s stored, whilst allowing on-demand access of said data.
What Is A Data Swamp?
OK, so if that’s a Data Lake, what’s a Data Swamp?
A Data Swamp is still a Data Lake… just a badly designed one, with little to no documentation to help support it that rarely, if ever, receives any maintenance.
That terrible design… that lack of documentation… that lack of support… it all makes it much harder, if not impossible to retrieve data, certainly in anything like a timely fashion.
If the data can’t be retrieved correctly then it can’t be analyzed. If it can’t be analyzed then you’re paying money to store useless data… congratulations! You’ve got yourself a Data Swamp.
What Are Data Lakes For?
Data Lakes have a variety of functions.
They can act as a repository for a random assortment of data (audio files, video files, documents, log files etc) ensuring they’re stored in a compliant way for audits. Data scientists and engineers within an organization can use them to access structured and unstructured data at the same time to help sandbox new analytical models they’re testing. They can also be used to help integrate data from both operational and transactional systems.
Whilst most users of business intelligence tools will be satisfied with a Data Warehouse, Data Lakes tend to be used more by auditors, specialized analysts and, as already mentioned, data scientist and engineers.
What’s The Difference Between A Data Warehouse And a Data Lake?
Data Warehouses are, to put it simply, organized. Data Lakes… less so.
Data warehouses are a mature and established technology that comes with a host of architecture and documentation that everyone agrees on.
The data housed in them is cleaned and structured, allowing for easy analytics.
However, large scale volume storage can be expensive.
Data lakes on the other hand are a much newer form of technology, and still suffer / benefit from an evolving standardized architecture blueprint.
They’re used to store raw (unprocessed) data in any format, so it could be structured or unstructured, in any format… text, audio, logs, images etc.
They offer much greater flexibility to data warehouses, with no data processing needed until the data is called upon, meaning storing the data is much cheaper.
Benefits Of Data Lakes
-
Easier Data Collection — Data Lakes make data collection and ingestion a much simpler process. Structured and unstructured data can be stored for a lower costs, only being processed when it’s required.
-
Greater ETL Support: Data lakes are great for offering ETL support as they can support real-time data streams with high velocity, allowing for multiple uses of the convergence of data.
-
Quicker Data Preparation: with a Data Like, data teams don’t need to log into various differing sources to access and prepare data, it’s all there, searchable in the lake. That functionality massively speeds up the time of data prep, allowing them to get on with more important tasks much quicker.
-
Increased Scalability: Data Lakes are able to use a distributed filing system, meaning they’re very scalable from the off.
-
More Collaboration, Less Silos: As all the information is in one place, data silos across an organization are done away with, allowing for a much more free flow of information internally.
How Can You Tell Your Data Lake Is Turning Into A Data Swamp?
One of the first (and biggest) signs will be your metadata (or lack thereof).
Metadata is there to describe your other data. Within a Data Lake it should be used as a tagging system to better enable search functionality.
It can also be used to tag where the data came from or when it came in.
Sounding familiar? Congratulations… you’ve probably got a Data Lake.
Not ringing any bells? Then be careful, you’re likely heading into some swampish territory.
Data Lakes are great for dumping data in if you’re not quite sure what to do with it just yet, you don’t want to transform it and pop it in a Data warehouse or you’re not quite sure how it fits in to your overall business strategy… yet.
But…
That doesn’t mean a Data Lake is there to capture every single iota of data that remotely touches your business.
In fact, one of the quickest ways of turning a pristine Data Lake into A Data Swamp is by filling it with irrelevant data.
It’s vital to know what kind of data you’re trying to capture as an organization and most importantly… why.
Once you do you can set up some parameters to only capture and store data that will be useful.
Data Governance is vital when handling any kind of data, whether it be structured or unstructured.
How to treat it, where to put it, who can see it, who can move it, how long it’s stored for… the last goes on and on.
No Data Governance on your Data Lake? Then you’ve probably got a Data Swamp (or soon will).
The lack of good Data Governance (or any in the worst case scenarios) often leads to Data Lakes being treated as a catch all for well… everything. They become bloated and unwieldy and that’s before we even enter the realms of regulation, with what data should be held, on who, for how long.
A Data Swamp can be a painful thing indeed if you suddenly find yourself being audited.
Automation is also important in preventing your new Data Lake turning into a Data Swamp.
Set your automation up right, and a lot of the above will be handled with no manual intervention needed.
If your Data Lake has a severe lack of automation though it means everything needs to be done manually, which is no one’s top priority, meaning you can end up with a data swamp before you even realize it!
Finally, it’s important to recognise that no one sets out to make a Data Swamp… it just sometimes happens.
One of the biggest steps you can take in avoiding it is to set a robust data cleaning strategy.
If your Data Lake (Swamp) is overrun with out of date, inaccurate, wrong or duplicated data then no one will trust or use it.
Nothing turns a Lake into a Swamp faster than dirty data.
Start your trial today
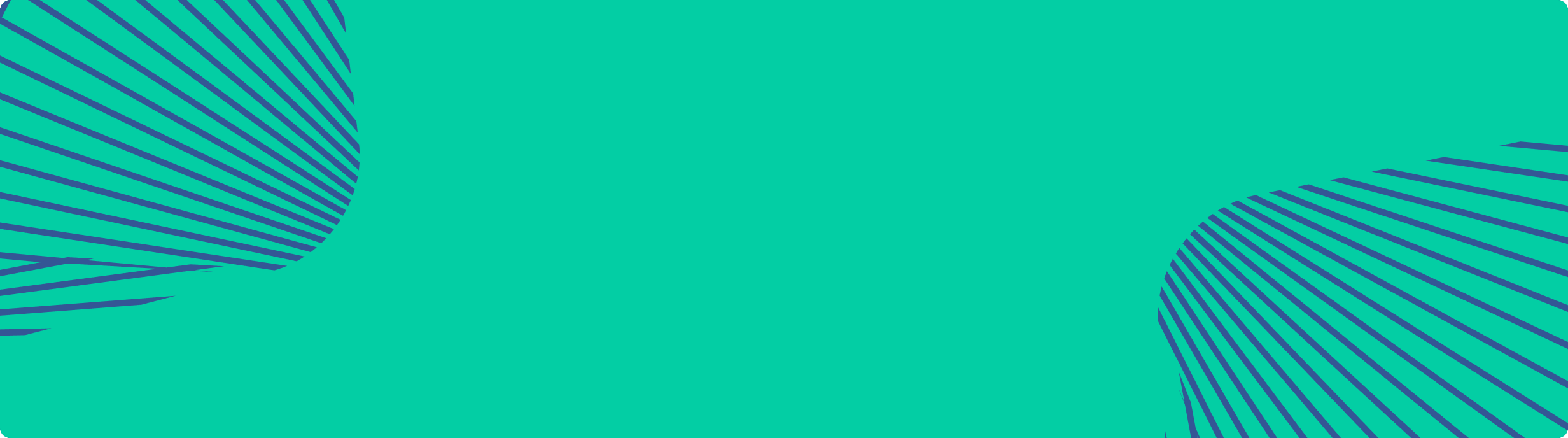