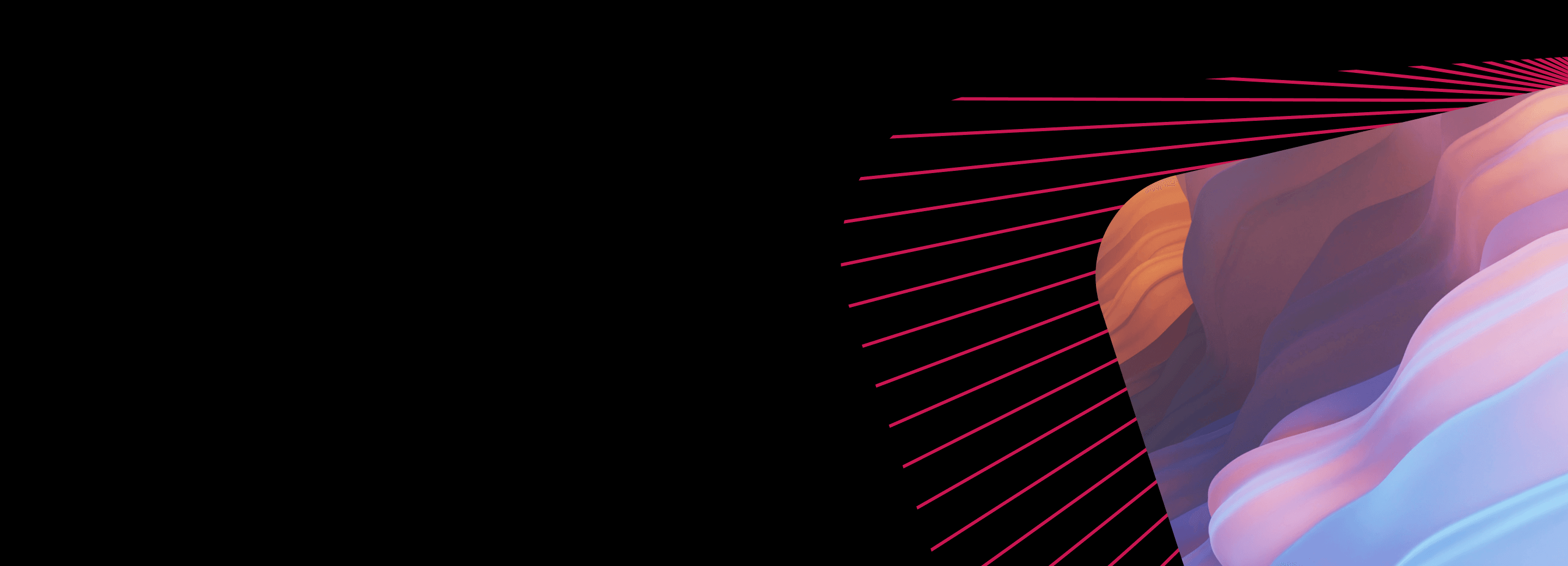
What is data quality? Importance, dimensions & impact
In today’s world, data plays a pervasive role. It is the foundation of information, empowering individuals and organisations with knowledge. Consequently, data has evolved into a valuable currency exchanged among various stakeholders.
The significance of data lies in its ability to enhance decision-making processes, greatly augmenting the prospects of success. The concept of data quality comes to our aid, facilitating the task at hand. Let us delve into the essence of data quality, exploring its defining characteristics.
What is data quality?
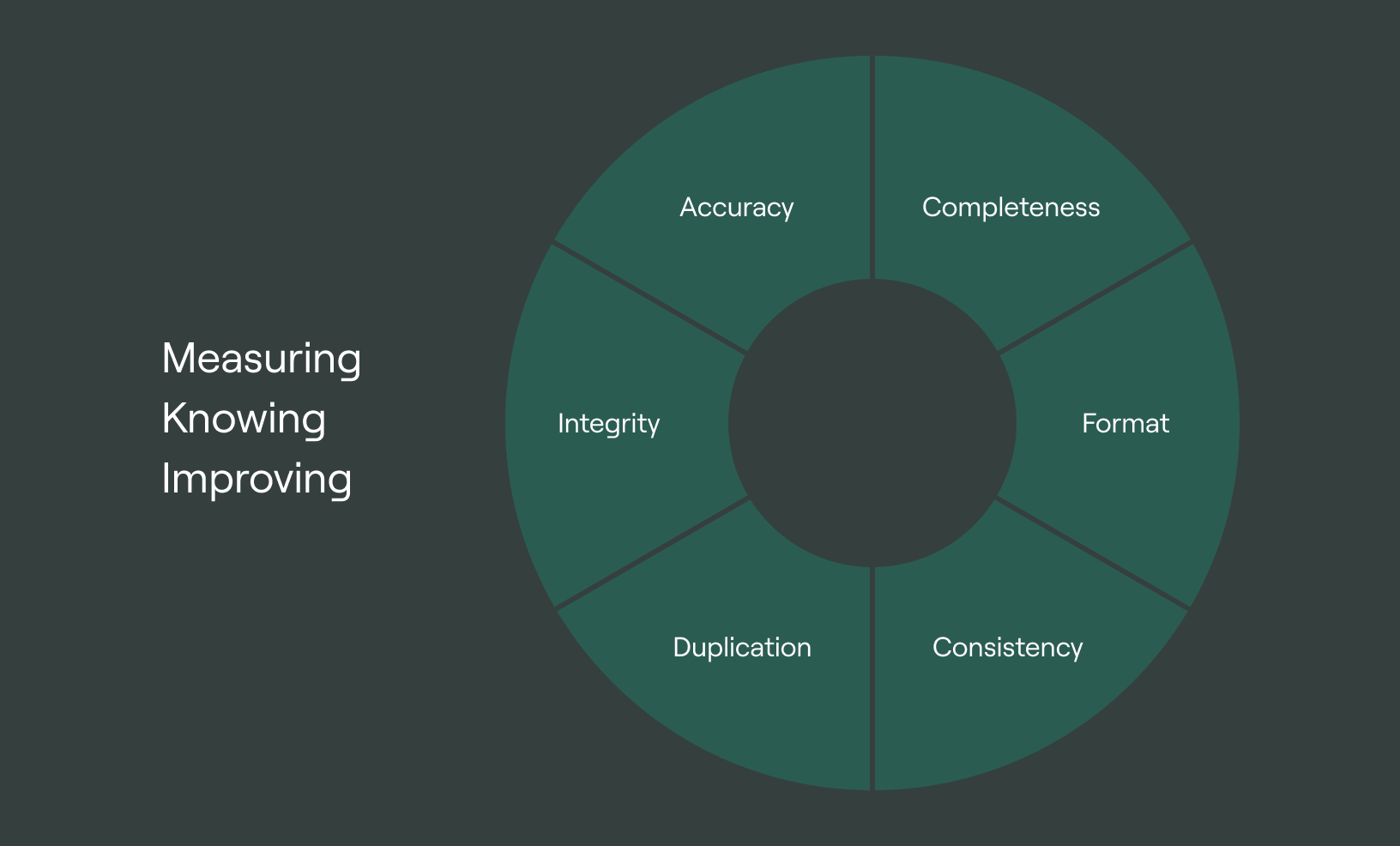
In this article, we’ll talk about:
- What is data quality?
- Why is data quality important today?
- Data quality dimensions
- Types of data quality issues
- What can cause data quality Issues?
- Impact of poor data quality
- Methods for ensuring data quality
- How to assess data quality?
- Benefits of data quality for business
- Challenges of ensuring large data quality
- Best practices for ensuring data quality
- Final words
Data quality plays a crucial role in data governance, ensuring that the data within your organization meets the required standards for its intended purpose. It encompasses the overall usefulness of a dataset and its seamless adaptability for processing and analysis in various contexts.
By effectively managing the dimensions of data quality, including completeness, conformity, consistency, accuracy, and integrity, you can enhance the effectiveness of your data governance practices, analytical endeavours, and initiatives in artificial intelligence and machine learning. This, in turn, leads to the generation of dependable and trustworthy outcomes.
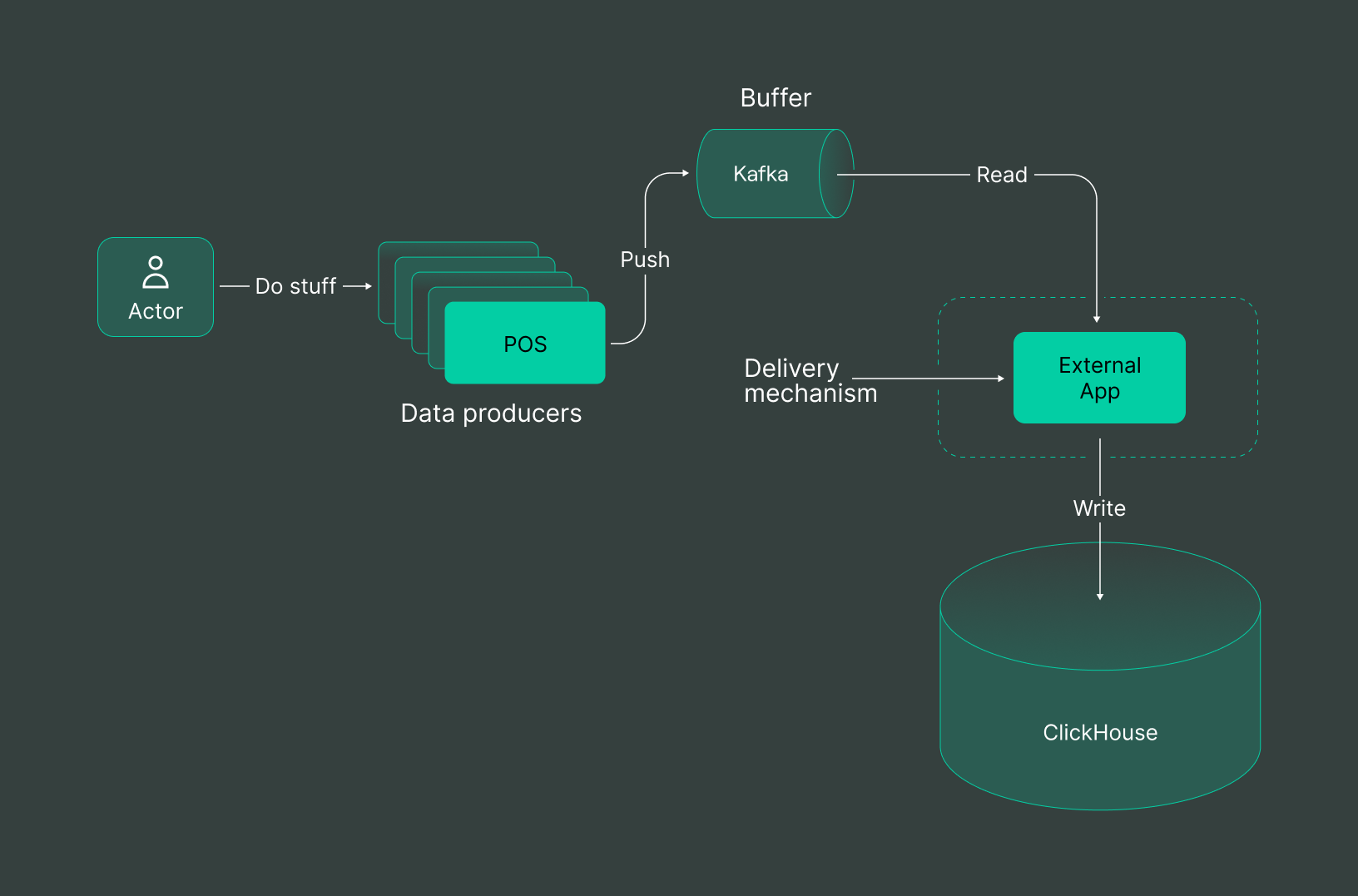
Why is data quality important today?
By prioritizing data quality, businesses can elevate their decision-making capabilities, enhance customer satisfaction, and reduce costs. The involvement of data management teams which includes data quality analysts, data scientists, data engineers, DevOps professionals, and analytics specialists is vital in ensuring the accuracy and completeness of data.
Data engineers are responsible for designing and constructing robust data pipelines, facilitating seamless data transfer between locations. DevOps engineers automate the deployment and management of software applications, contributing to the consistency and accuracy of data. Analytics specialists analyze data to uncover patterns and trends, empowering businesses to make informed decisions.
Data quality dimensions
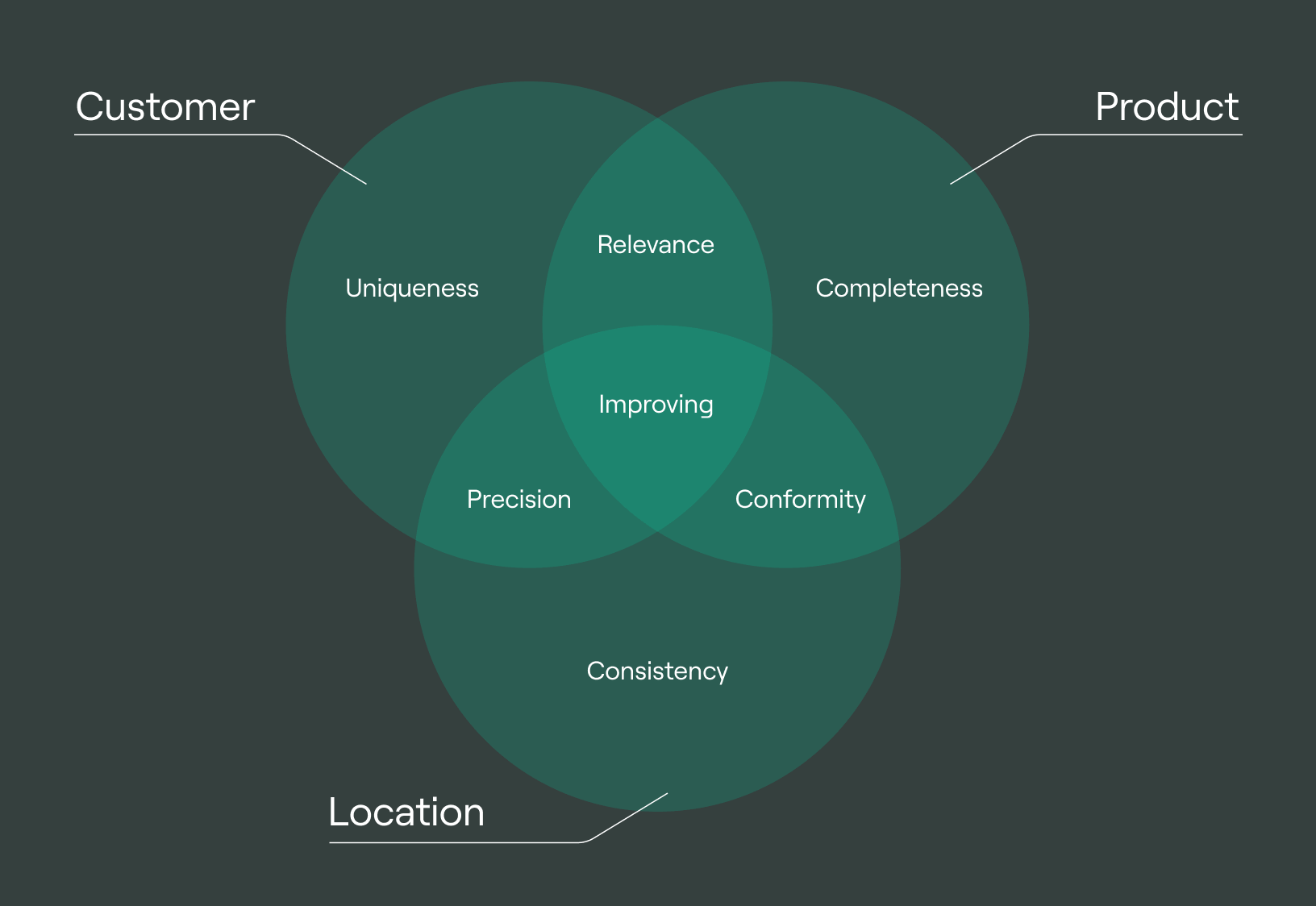
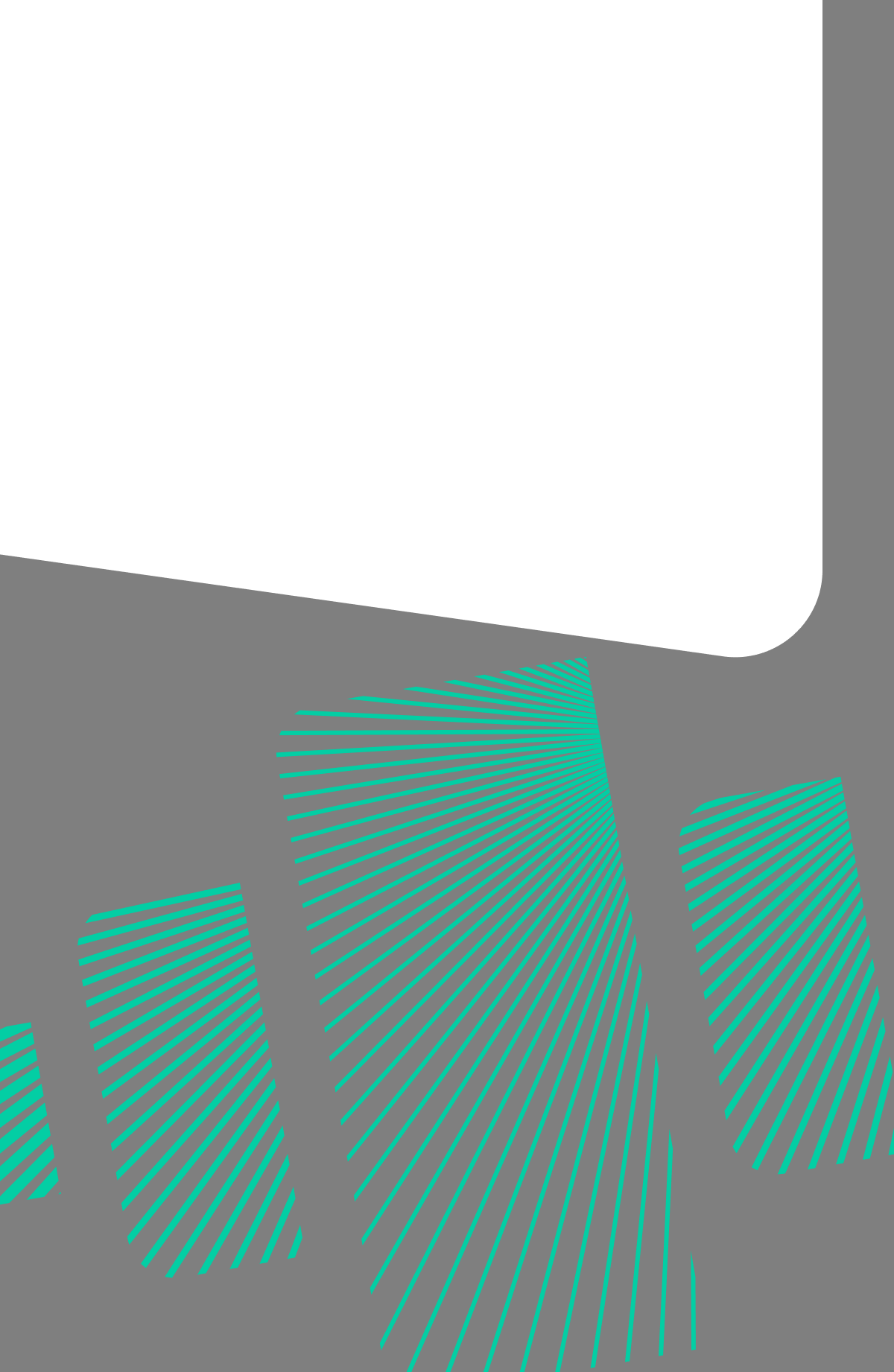
No-code ELT tool: Data Transfer
A cloud agnostic service for aggregating, collecting, and migrating data from various sources.
When focusing on data quality rules, the objective is to assess and improve various data quality dimensions. Here are the key performance indicators to be considered to measure data quality:
Accuracy: This is a fundamental aspect of data quality measures, ensuring that the data accurately represents the real-world objects or events it aims to portray. Evaluating accuracy involves comparing the values against a trusted information source to determine the level of agreement.
This comparison serves as a good data quality dimension to ascertain the reliability and correctness of the data.
Completeness: This refers to the degree to which the data includes all the essential records and values without any omissions or gaps. It guarantees that the dataset is thorough and contains all the necessary information needed to fulfil its intended purpose.
Consistency in data refers to the coherence and harmony of values obtained from various sources. It ensures that the data conform and there are no conflicting or contradictory data points within a record or message and across different values of a specific attribute.
It’s important to note that while data consistency is desirable, it does not automatically guarantee accuracy or completeness.
Timeliness: This relates to the currency and relevance of data. It emphasizes the need for data to be updated promptly, including real-time updates if necessary, to meet users' requirements in terms of accuracy, accessibility, and availability.
By ensuring timely updates, data remains current and aligns with the specific needs of users.
Uniqueness: This refers to the absence of duplicate records within a dataset, regardless of whether they exist in multiple locations. Each record within the dataset can be distinctly identified and accessed, both within the dataset itself and across various applications.
This ensures that each record is unique and eliminates any redundancy or repetition, facilitating efficient data management and retrieval.
Validity: Accurate data collection is essential, adhering to the organization’s established guidelines and criteria. Furthermore, the information must adhere to recognized formats and ensure that all data values are within the appropriate range.
Types of data quality issues
The consequences of inadequate data quality can span from minor inconveniences to complete business failure. Here are the types of data quality issues:
Inaccuracy
Data inaccuracy occurs when the information is incorrect or does not accurately represent the real-world context. For instance, an accuracy issue arises when a customer’s name is misspelt within a database.
Incompleteness
Incompleteness refers to the state of data being inadequate due to the absence of crucial information. For instance, an incompleteness issue arises when a customer’s address is not included in a database, leaving a gap in the required data.
Inconsistency
Inconsistent data is a common challenge in data quality. This occurs when it lacks uniformity in format or fails to adhere to predefined rules. For instance, if certain customers' phone numbers are recorded in the format (123) 456-7890 while others are in the format 123-456-7890, it signifies a consistency issue, as there is a lack of standardized formatting across the dataset.
Duplication
Data duplication arises from various causes, including human error, system errors, and data migration processes. Identifying and addressing these issues is crucial to ensure data integrity and avoid complications associated with redundant and fragmented information.
What can cause data quality issues?
These issues can be caused by multiple factors, including human error and entry errors. To keep track of and resolve the concerns, they should be recorded in a thorough data quality issue log. Here are a few factors that can affect data quality:
Human error
Human error is one of the data quality issues raised, including data entry errors like typos and incorrect formatting, as well as errors in judgment, such as relying on unverified assumptions. Such mistakes can substantially impact the accuracy and reliability of the data.
Data entry mistakes
Data entry errors can occur when information is manually entered into a system, resulting from fatigue, distractions, or inadequate training. These factors can compromise the accuracy and integrity of the entered data, underscoring the significance of attentiveness and thorough training in data entry procedures.
System or hardware failures
Some data entry errors can occur during the manual input of information into a system. Factors such as fatigue, distractions, and lack of training can contribute to these mistakes. These errors harm the accuracy and reliability of the entered data, underscoring the need for attentiveness and adequate training in data entry tasks.
Impact of poor data quality
Here are some of the impacts poor quality data can create:
Reduced decision-making effectiveness
Relying on flawed or incomplete data for business decisions can lead to overlooking crucial information and this goes against the business rules. For instance, if your out-of-home ads drive most conversions and brand awareness, but an incomplete attribution model misguides resource allocation to less effective media channels, your ROI may suffer.
Increased costs
Poor data quality is costing organizations trillions of dollars. Nearly half of all newly acquired data is inaccurate, which can have a negative impact on businesses. According to MIT, bad data can cost businesses up to 25% of their total revenue. These statistics highlight the importance of data quality.
Decreased customer satisfaction
Bad data can harm not only your advertising budgets but also your customer relationships. When inaccurate data leads to targeting customers with irrelevant products and messaging, it can quickly sour their perception of the brand. This can result in customers opting out or disregarding future communications. It highlights the need for accurate data to ensure positive customer experiences and engagement.
Methods for ensuring data quality
Here are some data quality solutions to enhance the quality of data:
Data profiling
Data profiling involves gathering and examining data to gain insights into its characteristics. This includes detecting data quality problems like missing values, incorrect data types, and duplicate records. By conducting data profiling, organisations with corporate data assets can pinpoint areas that require enhancements in data quality.
Data cleansing
Data cleansing refers to the practice of identifying and rectifying data errors. This can be achieved using digital asset management systems or by utilizing specialized data cleansing tools. Ensuring good data quality relies significantly on data cleansing, as it plays a crucial role in enhancing the accuracy and dependability of the data.
Data validation
Data validation involves examining data to verify its accuracy and completeness. This verification can be carried out through manual checks or by utilizing data validation tools. The purpose of data validation is to ensure that the data is suitable and reliable for its intended purpose.
Master Data Management
Master Data Management (MDM) is a practice that focuses on maintaining the accuracy, consistency, and completeness of essential master data across an organization. Master data refers to the fundamental information about key entities like customers, products, and suppliers.
By implementing MDM, businesses establish a centralized and reliable data source of truth for this data. This approach enhances customer data integration, decision-making, minimizes expenses, and enhances customer satisfaction by ensuring the enterprise data is consistent and reliable.
Product master data, location master data, and party master data which are all kinds of MDM are vital for data quality. Accurate product data ensures reliable information, consistent location data enables accurate spatial analysis, and comprehensive party data enables precise customer segmentation and personalized services, all contributing to improved data quality.
Data governance
Data governance encompasses a series of procedures and guidelines aimed at guaranteeing the quality, usability, and security of data within an organization. A data governance framework ensures data is handled consistently, adhering to relevant regulations and internal policies.
Additionally, data governance safeguards data from unauthorized access, misuse, disclosure, interruption, alteration, or loss. By implementing robust data governance practices, organizations can maintain data integrity, facilitate compliance, and mitigate potential risks associated with data management.
Data matching
Data Matching is the process of comparing data elements from multiple sources to find potential matches. It helps identify duplicate records, link data from different systems, and reconcile data discrepancies. Data matching techniques enhance accuracy, streamline integration, and ensure system consistency.
Some other methods of ensuring data quality include data protection regulations. This plays an important role in improving data security. Also, location master data.
How to assess data quality?
Data quality assessment ensures accurate, reliable, and valuable data. It involves identifying multiple data quality variables such as accuracy, completeness, and consistency. On a data quality dashboard, techniques like data profiling, data cleaning, and data validation are displayed.
These techniques are frequently used by organisations to check the quality of their data and increase its correctness and dependability. Objective data quality assessments help organisations identify future data quality issues, correct errors, and ensure that data is suitable for its intended use.
A good data set is accurate, complete, consistent, timely, and relevant. The data quality indicators should have the same data values. These subjective data quality metrics ensure correctness, inclusiveness, harmony, up-to-date information, and direct applicability, making the data set valuable and reliable.
Benefits of data quality for business
Here are some of its benefits as it is essential for business.
Improved decision-making
By ensuring data quality, businesses can enhance their decision-making processes. Accurate and reliable information allows businesses to identify trends, make predictions, and develop effective strategies. For instance, a gaming company can leverage data quality efforts to determine the popularity of different games among players, identify underperforming games, and prioritize improvements.
This valuable insight enables the company to make informed decisions regarding game development, marketing efforts, and customer support, ultimately leading to better outcomes.
Increased revenue and profits
Data quality is crucial for businesses to drive revenue growth and profitability. By improving data quality, businesses can enhance their marketing, sales, and customer service efforts. For example, a gaming company can utilize data quality to target marketing campaigns more effectively, increasing game sales and improving customer engagement.
Better customer experience
Data quality plays a crucial role in enhancing the customer experience for businesses. By ensuring high data quality, businesses gain insights into customers' needs and preferences, enabling them to personalize the customer experience, improve customer service, and foster customer loyalty.
For instance, a gaming company can leverage data quality to track gameplay data, identify customer preferences, and deliver personalized experiences.
Enhanced reputation and trust
Customer master data in data quality ensures accurate, reliable and consistent information about customers which builds reputation and trust. For instance, a gaming company can utilize data quality to monitor gameplay data, identify customer preferences, and deliver personalized experiences.
Challenges of ensuring large data quality
Ensuring data quality poses various challenges for businesses. Some of these challenges include:
Data governance and ownership
In organizations, data governance and ownership are crucial for maintaining data quality, usability, and security. However, establishing clear data governance and ownership policies can be challenging in large organizations.
This can result in data quality issues due to inconsistent standards among departments and a lack of accountability for complete and consistent data. It is important to address these challenges by implementing effective data governance frameworks and clearly defining assigned data ownership to ensure data quality across the organization.
Lack of standardization
Lack of standardization in data formats and systems can hinder data quality in large organizations. With data scattered across different systems using varied formats and standards, integrating and reconciling the data becomes challenging. This lack of data standardization can lead to data quality problems like inconsistent product codes or conflicting customer definitions.
As a result, data transfer between systems may introduce errors, undermining the accuracy and reliability of the data. Standardized data formats and robust data integration processes can help address these challenges and enhance data quality.
Complex data environments
In large organizations, managing data quality can be challenging due to complex data environments. Data is often stored in diverse locations, including on-premises and the cloud, making it difficult to track data movement and ensure its up-to-date status.
Balancing data quality with data availability
Striking a balance between data quality and data availability can be a challenge. There are instances where businesses may need to prioritize making data available to users, even if it’s not entirely accurate. Finding the right equilibrium between data quality and data availability can be tricky, as it requires careful consideration.
Best practices for ensuring data quality
The data quality improvement process ensures that high data quality is achieved. Here are some recommended approaches to maintaining data quality:
Establish data quality standards
It is essential to create clear standards for data quality that outline the characteristics of reliable data. All relevant parties within the organization should collectively agree upon these standards. To ensure consistent data quality, they should encompass key aspects like accuracy, completeness, timeliness, consistency, and relevance.
Ensure data accuracy at the source
Emphasize accurate data collection and entry from the beginning to avoid costly and time-consuming error corrections. Use data entry formats, conduct regular audits, and provide training to data entry staff to maintain data accuracy at the source. Data stewards are mostly responsible for this.
Implement automated data quality checks
Employing automated data quality checks is essential for detecting and resolving issues in data quality. Regularly running these checks helps ensure ongoing data quality. Numerous data quality tools are accessible for automating these checks and maintaining high-quality data.
Continuously monitor data quality
It is important to continuously assess data quality to ensure it aligns with the organization’s standards. This can be achieved through periodic data quality reviews and the implementation of a data quality management system. An effective data quality management system comprises a series of processes and procedures designed to uphold high-quality data.
Final words
Data quality is essential for achieving higher operational efficiency, cost savings, and a solid KPIs foundation for decision-making based on reliable data. When data is of high quality, businesses can effectively manage their data analytics operations, even in the face of rapidly increasing data volumes.
Data integrity is vital for businesses and organizations, ensuring data accuracy, completeness, and consistency throughout its lifecycle. This integrity has several benefits, including improved decision-making, increased operational efficiency, and regulatory compliance.
Ensuring data quality can be challenging, but the investment of time and effort is well worth it for the long-term success of your business.
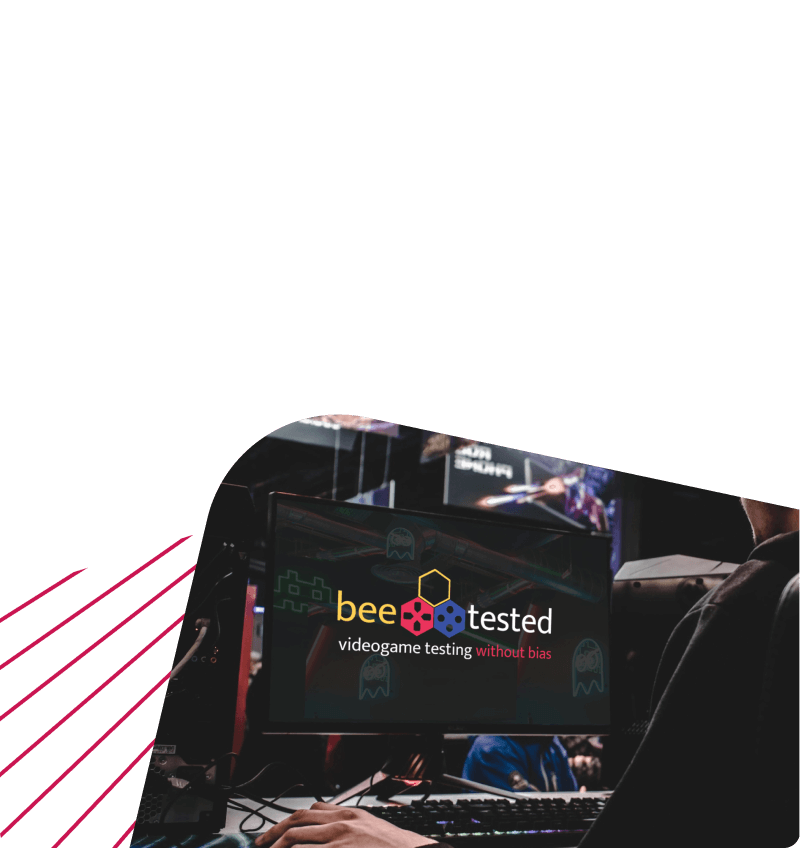
Beetested analyze millions of gamers emotions with DoubleCloud’s Managed ClickHouse solution
Saving days of work by creating and embedding analytics directly from the data in their ClickHouse clusters
Frequently asked questions (FAQ)
What is the main goal of data quality?
What is the main goal of data quality?
The primary objective of data quality is to guarantee the accuracy, completeness, consistency, relevance, and timeliness of data. This is crucial because data serves as the foundation for decision-making, and any inaccuracies or gaps in the data can result in poor decision outcomes.
How do you measure the quality of data?
How do you measure the quality of data?
What are the components of data quality?
What are the components of data quality?
How do you improve data quality?
How do you improve data quality?
Start your trial today
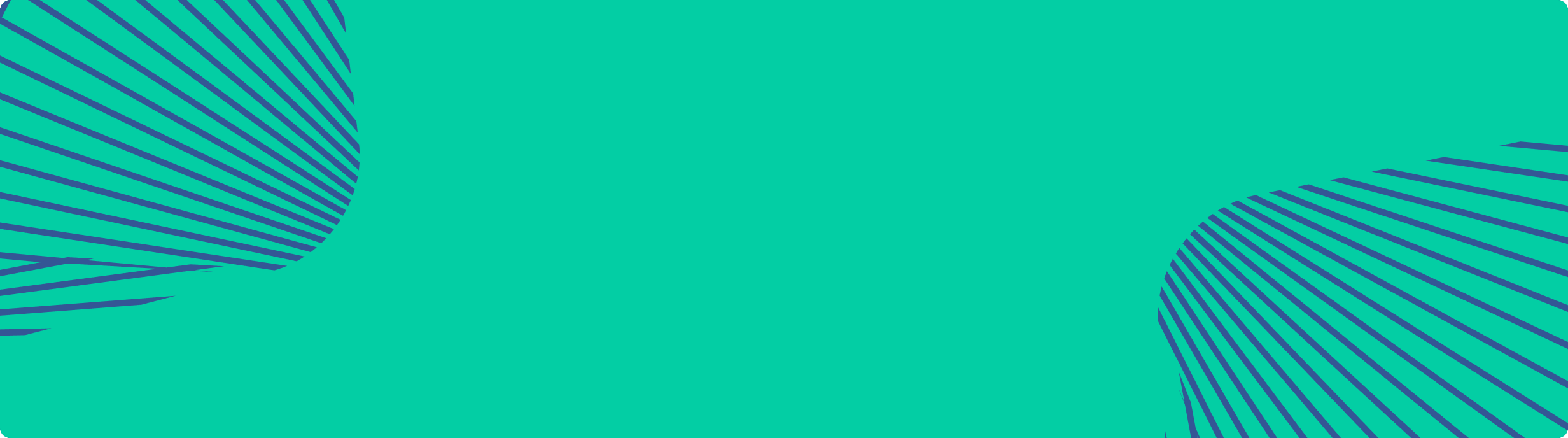